
The Exponential Growth of Data
The past decade has seen an explosion in the amount of data generated and collected by organisations. Thanks to digital technology like cloud computing, social media, mobile devices and the Internet of Things, we now create a staggering 2.5 quintillion bytes of data every single day. To put that into perspective, 90% of all the world's data has been created just in the last two years.
This relentless growth presents both opportunities and challenges for organisations. On one hand, this data holds tremendous potential value - it can reveal insights about customers, optimise operations, predict trends and more. However, organisations often struggle to actually make use of their massive data stores. The volume is simply too great for humans to comprehend and traditional analytics methods fall short. Most companies today are data-rich but insights-poor. Critical business questions go unanswered because organisations lack the ability to glean meaning from their overwhelming volumes of data. Valuable patterns and correlations get lost in the deluge.
Defining Data Visualisation
Data visualisation refers to the graphical representation of information and data. It involves creating visual depictions like charts, graphs, maps, and diagrams to communicate insights, patterns, and trends within data sets. The main goal of data visualisation is to make complex data easier for audiences to understand and interpret.
Effective data visualisations optimise the ability to comprehend large amounts of data quickly. Viewers can identify relationships, differences, and meanings that may otherwise be difficult to interpret in tables, reports, or dashboards full of numbers. Well-designed visuals act as tools for analysis, communication and discovery.
There are many types of data visualisations, each with their own strengths and purposes:
Bar charts - Useful for comparing values across different categories or time periods
Line graphs - Ideal for showing trends and changes over time
Pie charts - Provide a simple proportional breakdown in a single data set
Scatter plots - Reveal the relationship between two variables through their intersection
Heatmaps - Use colour to represent values and intensity of metrics
Geospatial maps - Display location-based data like demographics, traffic, and weather
The key is selecting the right visual to match the specific data type and relationships you want to highlight. Effective data visualisation optimises comprehension, highlights patterns and provides actionable insights from complex data.

Complex Data and Its Challenges
The volume of data being generated today is exploding at an exponential rate. With the proliferation of smart devices, social media, ecommerce and more, organisations are accumulating vast amounts of complex data on a daily basis. But what exactly constitutes complex data?
Complex data refers to large datasets with intricate structures, multiple data types, and/or a high degree of variability. For example, clickstream data from website interactions contains different data types like page views, timestamps, locations etc. Patient healthcare records include demographics, diagnoses, medications, labs, images and more. Complex data has the following key characteristics:
High volume - contains a large number of records or data points
High dimensionality - comprises multiple attributes and dimensions
Heterogeneous data types - includes structured, unstructured and semi-structured data formats
Temporal nature - changes over time requiring trend analysis
Interconnectedness - data elements have multiple relationships across domains
The intricacy of complex data makes it very difficult to interpret, analyse and glean actionable insights from it. Traditional data analysis techniques fall short in handling the scale, diversity and interconnectedness of complex data. The absence of contextual meaning causes complex data to lack descriptive value without deeper examination.
Some common sources of complex data include:
Transactional data like purchases, logins, clicks etc.
Internet of Things (IoT) sensor data from smart devices
Social media content and interactions
Satellite imagery, climate and geospatial data
Genomic data from biological systems
Network and graph data representing connections
Unstructured data like images, video, audio, text documents
Complex data underpins many of the technologies and applications we use daily. But unlocking its potential requires moving beyond surface-level analysis to find meaningful patterns and actionable intelligence hidden within.
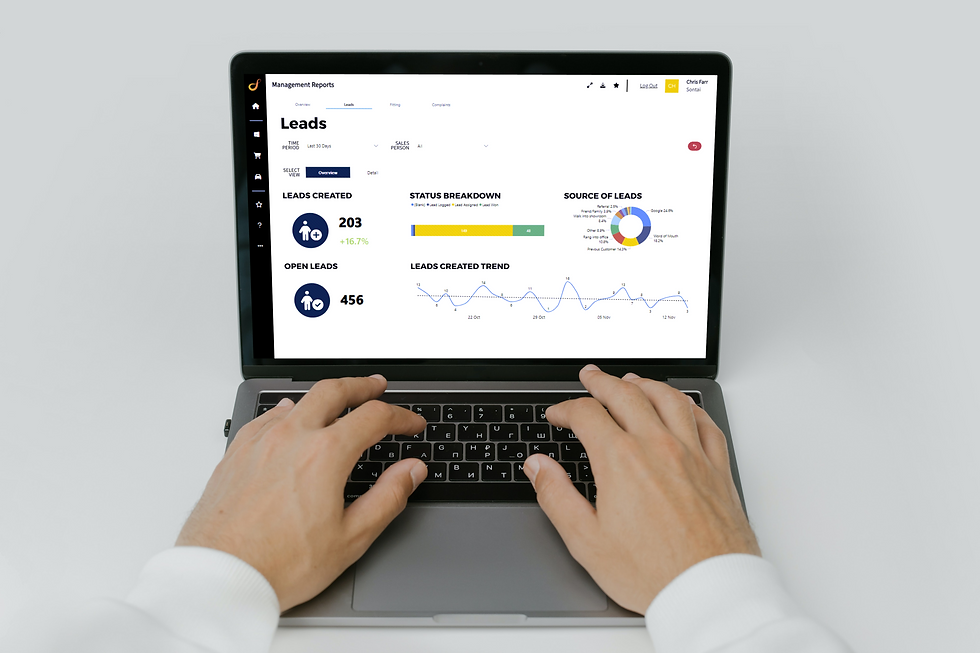
Principles of Effective Data Visualisation
Creating insightful and powerful data visualisations requires following certain design principles and best practices. Some key principles for effective data visualisation include:
Simplicity is Key
The most effective visualisations are simple and focused. Eliminating all non-essential elements allows viewers to quickly grasp the key insights. A simple design also makes the visualisation aesthetically pleasing. Cluttered and overly complex designs fail to communicate key messages.
Clarity Above All
Every element in a visualisation should have clarity of purpose. Use labels, annotations and highlighting techniques to maximise clarity. Remove ambiguous elements that create confusion. The purpose and message of the visualisation should be immediately apparent to the viewer.
Leverage Visual Encodings
Visual encodings like colour, size, shapes and position allow viewers to efficiently process information. For example, varying bubble size instead of colour to denote magnitude. Using appropriate visual encodings for data types improves comprehension.
Maintain Consistency
Using consistent visual mappings, colour schemes, fonts, styles and layouts allows easy comparison of different data views. Consistency aids memorability and learning. Vary visuals only when it serves a specific purpose.
Convey a Story
Organise visualisations in a logical narrative flow that connects facts to reveal insights. Use annotations and highlighting to guide the viewer. Craft compelling headlines and summaries that capture key messages.
Optimise Readability
Strive for visual hierarchy, logical layouts, and effective use of white space. Avoid cramped and cluttered designs. Allow easy path for the eyes to flow across visual elements. Enable effortless data comparisons.
Keep Iterating and Improving
Creating insightful visualisations often requires plenty of experimentation and iterations. Continuously refine designs to make the complex simple and amplify key insights. The most brilliant ideas start as rough sketches.
Following proven design principles elevates data visualisation from merely presenting facts to revealing powerful and actionable insights through visual storytelling.

Data Visualisation Tools and Technologies
Data visualisation tools provide the software capabilities to process, analyse, and visualise data in intuitive ways. With the growing popularity of data visualisation, many tools have emerged with different features and capabilities. Selecting the right tool depends on understanding the strengths and limitations of leading options.
Comparison of Tools
When comparing the leading data visualisation tools, some of the factors to consider include:
Ease of use - Is the tool easy to use and set up or does it specialist expertise.
Visual appeal - Do the outputs fit with the key principles above, or are the reports generated hard to read and interpret.
Data connectivity - Can you connect to all your data?
Customisation - How easy is it to customise what you need?
Cost - How does the cost change as you add more data or more users?
Selecting the Right Tool
Consider the key factors below when choosing a data visualisation tool:
Audience - Is the tool ready for non technical report users? Or does it require large amounts of training to get people up and running?
Data sources - Pick tools that can easily connect with your data sources like SQL, cloud storage, online applications etc.
Budget - Can you easily manage your budget? Or are there hidden costs that make it harder to understand the true cost of your tools?
By evaluating leading options based on these criteria, you can determine the best tool for your specific data visualisation needs. The key is matching business objectives with tool capabilities.
Introducing Sontai
Sontai offers a powerful prebuilt BI solution designed specifically for small and midsize enterprises (SMEs). Our intuitive cloud platform combines key capabilities like data visualisation, advanced reporting and data integration in one unified interface.
Sontai aims to make enterprise-grade BI accessible for organisations without the IT resources of large corporations. The preconfigured dashboards and reports allow stakeholders across the business to self-serve the insights they need in minutes without relying on IT or data experts.
Key features and advantages include:
Library of pre-built templates for multi-channel sales, category, finance, automotive and more.
Automated data integration from multiple sources.
Role-based access and sharing controls.
Cloud deployment for ease of access.
Sontai's prebuilt BI is designed for the needs of specific departments and industries. It has delivered tremendous value to customers across consumer goods, retail, automotive, waste management and more.
For example, a consumer goods brand used Sontai's prebuilt sales analytics template to gain insights into their retail channels, product, customer and promotional performance. By optimising promotions based on data-driven insights, they achieved a +12% increase in marketing ROI within months.
Check out how Sontai compares to other leading data visualisation and reporting tools
Best Practices for Creating Effective Data Visualisations
To create insightful data visualisations that clearly communicate key information, it's important to follow best practices when working with data. Here are some tips:
Preparing and Cleaning Data
Ensure data is accurate, complete, and consistent. Fix any errors, outliers, or duplicate entries.
Filter out unnecessary data fields to simplify the dataset. Remove variables that are irrelevant to your analysis.
Aggregate data points if needed to avoid cluttering the visualisation. Derive new metrics, averages, percentages etc.
Normalise units of measurement so data can be combined effectively. Convert currencies, units of time, distances etc.
Choosing Optimal Visualisations
Select visualisations based on data type: bars for categorical data, lines for trends over time, scatter plots for correlations etc.
Consider audience and context. Use simple charts for general audiences and more complex ones for technical users.
Limit the number of variables per chart. Too many data points create clutter.
Use colour judiciously to highlight patterns but avoid decorative effects.
Effective Design Principles
Eliminate clutter and unnecessary elements. Stick to the most impactful data points.
Draw attention to the point of focus through layout, positioning, colours.
Maintain integrity of the data. Do not exaggerate or alter data to emphasise trends.
Keep text short and descriptive. Label properly and use captions sparingly.
Maintain consistency in visual treatments across charts for intuitive understanding.
By preparing data correctly, choosing optimal visualisations, and employing effective design principles, you can create data visualisations that clearly communicate insights from complex data sets. This drives informed decision making and impact.
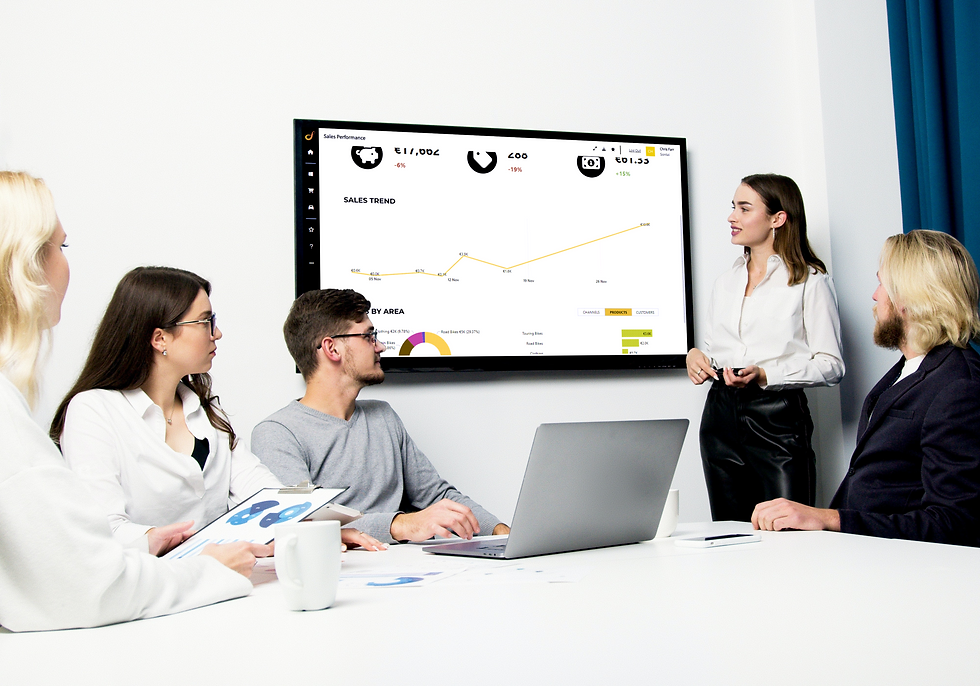
The Future of Data Visualisation
Data visualisation is a rapidly evolving field driven by emerging technologies and user needs. As data continues to grow exponentially, new techniques and tools are required to make sense of complex information. Several key trends are shaping the future of the data visualisation landscape:
Emergence of Augmented and Virtual Reality
Immersive technologies like augmented reality (AR) and virtual reality (VR) are finding their way into data visualisation. AR overlays data on real-world environments while VR creates simulated 3D environments for interacting with data. This brings data to life in innovative ways and enhances data exploration. AR/VR expand the possibilities for data storytelling beyond 2D screens.
Growth of Natural Language Generation
Natural language generation (NLG) automatically converts data into written analysis and insights in plain language. This allows data visualisations to be accompanied by customised textual narratives tailored to different audiences. NLG makes data insights more consumable while retaining objectivity.
Mainstreaming of Interactive Dashboards
Interactive dashboards allow users to explore data by applying filters, clicking data points to drill down into details, and manipulating charts in real-time. As self-service analytics gains popularity, interactive dashboards are becoming critical for data democratisation across organisations. Their flexibility and intuitiveness make them indispensable analysis tools.
Embedding Advanced Analytics into Visuals
Data visualisation is moving beyond basic charts and graphs. It increasingly incorporates advanced analytics like statistical models, predictive algorithms, and machine learning techniques to uncover hidden insights. Visualising these complex analytics makes them more interpretable and actionable.
Focus on Automation
Automating data visualisation workflows will accelerate creation and enable real-time updates. Auto-visualisation features in tools generate initial charts from data, allowing faster iterations. Cloud-based platforms enable assembling dashboards using APIs instead of manual work. These automation capabilities enhance productivity.
Data visualisation will continue evolving in line with emerging technologies to meet rapidly changing business needs and scale to growing data volumes. It is poised to become even more immersive, intelligent, and integral to data-driven decision making. The possibilities to make data insights more intuitive and accessible are endless.
Kommentarer